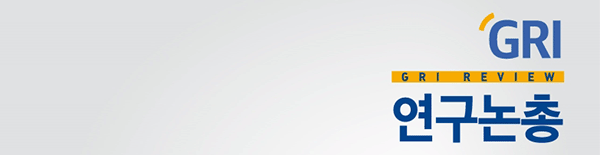
Exploring the Impact of Trip-Chaining on Commute Mode Choice in the U.S.
Abstract
This study aims to explore trip-chaining and its impact on commute mode choice using data from the 2017 U.S. National Household Travel Survey (NHTS). This study defines trip-chaining as a complex work tour where non-work activities (i.e., shopping/family errands, medical services, school/daycare/religious activity, social/recreational activity) are conducted on the journey between home and work. We first analyze the trip-chaining characteristics by simply assessing the descriptive statistics. We then employ a multinomial logistic regression model to examine the determinants of commute mode choice and the impact of trip-chaining. Key findings from our empirical analyses are as follows. First, women make more non-work trips than men while commuting, and men and women have more chained trips after work. Second, they usually have chained trips to drop off/pick up other individuals, buy meals, and buy goods while commuting. Third, women are more likely to prefer driving alone over public transit and walking/cycling. Fourth, urban neighborhoods are more likely to make individuals use public transit, walking/ cycling, and carpooling. Lastly, trip-chaining can act as a barrier to public transit and walking/ cycling.
초록
본 연구의 목적은 2017년 미국 가구통행실태조사자료(NHTS)를 활용하여, 트립체인(Trip-Chaining)의 특성과 트립체인이 통근수단 선택에 미치는 영향을 탐구하는 것이다. 본 연구는 트립체인을 집과 직장을 연결하는 통근통행 중에 비업무 목적의 통행 (즉, 쇼핑/가사 업무, 의료, 돌봄/종교 활동, 기타 사회활동 등)이 포함되는 복잡한 통근통행으로 정의한다. 본 연구는 먼저 기초통계분석을 통해 트립체인의 특성에 대해 분석하고, 다항 로지스틱 회귀분석 모형을 이용하여 통근수단 선택에 미치는 요인과 트립체인의 영향에 대해서 분석한다. 연구의 결과는 다음과 같다. 첫째, 여성은 남성보다 더 많은 트립체인을 가지며, 남성과 여성은 출근할 때 보다 퇴근 후 통행에서 더 많은 비업무 통행을 수행한다. 둘째, 그들은 누군가를 태우거나 내려줄 때, 음식을 구매할 때, 물건을 구매할 때 주로 트립체인을 갖는다. 셋째, 여성은 대중교통, 보행/자전거 보다는 단독 자가용을 선호한다. 넷째, 도시지역에 거주하는 통근자들은 대중교통, 보행/자전거, 카풀(carpool)을 더 선호한다. 마지막으로, 트립체인은 통근통행에서 대중교통과 보행/자전거 수단 선택에 장벽으로 작용할 수 있다.
Keywords:
Commute Behavior, Trip-Chaining, Commute Mode Choice, U.S. National Household Travel Survey (NHTS), Multinomial Logistic Regression Model키워드:
통근통행 행동, 트립체인, 통근수단 선택, 가구통행실태조사, 다항로지스틱회귀모형Ⅰ. Introduction
A continuing challenge for transportation planners and policymakers is to identify motivators or barriers to reducing the dominance of the automobile, especially during peak traffic periods (Gordon et al., 1988; Hensher and Reyes, 2000). This is because outcomes of automobile usage, especially vehicle miles traveled (VMT), are associated with increased greenhouse gas (GHG) emissions, traffic congestion, and energy consumption (Golob and Hensher, 2007; Chen and Akar, 2017). The success of their effort is significantly related to the flexibility to change commute mode; that is, they have encouraged individuals to use public transit, active transportation (walking or cycling), and carpooling (Schmöcker et al, 2010; Akar et al., 2016). Therefore, it is important to understand factors affecting individuals’ commute behavior.
Individuals’ commute behavior has been becoming more complicated as socio-demographics of the society are undergoing significant changes (Kwon and Akar, 2022). Over the past decades, changing roles of women in society have made women enter the workforce in greater numbers, and increases in female commuters, vehicle owners, and growth in the number of two-earner households have accompanied this trend, which has led to changes in commute patterns (Rosenbloom, 2004; Crane, 2007; Fan, 2017). One such characteristic is trip-chaining, where non-work activities are conducted on the journey between home and work. As women have spent more time at work, they have less time performing non-work activities such as shopping and family errands. Inevitably, this trend leads to changes in an individual’s commuting behavior. For instance, all household members have to share non-work activities and perform them while commuting (McGuckin et al., 2005; Kwon and Akar, 2022).
Trip-chaining is a growing phenomenon and is becoming a significant part of some workers’ daily travel (McGuckin et al., 2005; Noland and Thomas, 2007). For instance, McGuckin et al. (2005) argue that the work trip has become more complex as workers incorporate personal, household, and child-care activities into their commutes. They find that more non-work trips, such as shopping and family errands, have been pushed into work trips. A great deal of attention should be paid to the impact of trip-chaining on commute behavior, as integrating non-work activities into commuting can increase peak-hour congestion and travel costs (Hensher and Reyes, 2000; Schmöcker et al., 2010; Duncan, 2015). Although actual commuting often involves secondary activities, limited work has been done to take into consideration trip-chaining and its impact on commute mode choice (Schmöcker et al., 2010; Chen and Akar, 2017). Within this consideration, this study explores trip-chaining characteristics and their impact on commute mode choice.
To understand whether trip-chaining is a barrier to the use of public transit, active transportation (walking/cycling), and multimodality (more than one mode), we utilize detailed individual level data from the 2017 National Household Travel Survey (NHTS). This study defines trip-chaining as a complex work tour where non-work activities are conducted on the journey between home and work. We first analyze the trip-chaining characteristics by simply assessing the descriptive statistics. We then employ multinomial logistic regression model (MLM) to examine the determinants of commute mode choice and the impact of trip-chaining.
This study is organized as follows. The next section reviews the literature on trip-chaining and commute mode choice. In section 3, we present our data, descriptive statistics, and analysis methods. In section 4, we present model estimations and interpretations. In section 5, we conclude with important findings, recommendations, and limitations of this study.
Ⅱ. Literature review
This section starts with the definition of trip-chaining, followed by the literature on the characteristics of trip-chaining.
1. Definition of trip-chaining
Although there is no formal agreement on the definition of a chain or how to systematically identify one in a dataset (McGuckin et al., 2005; Primerano et al., 2008), trip chaining refers to the propensity to link spatially and temporally interrelated trips within a multi-stop tour or journey (Adler and Ben-Akiva, 1976; Lee et al., 2007; Schmöcker et al., 2010).
Trip-chaining has been examined using two different concepts: one is a tour-based concept, and the other is a stop-based concept. Some studies are based on the tour-based concept, which is defined as a series of trips that begin and end at home so as to engage in specific activities (for instance, Lee et al., 2007; Ye et al., 2007). For instance, based on the tour-based concept, Stratham and Dueker (1995) distinguish between simple journeys involving a trip from home to a given destination and then returning home and complex journeys involving a sequence of more than two trips that begin and end at home. Based on Stratham and Dueker’s (1995) research, Hensher and Reyes (2000) use seven trip chain alternatives: simple work, complex to work, complex from work, complex to and from work, complex at work, simple non-work, and complex non-work.
Others use the stop-based concept, which is based on adding an activity to a standard home-based trip or non-home-based trip (for instance, McGuckin and Murakami, 1999; McGuckin et al., 2005; Noland and Thomas, 2007). For instance, McGuckin and Murakami (1999) define the stop as an intermediate stop of a tour. For example, if a person leaves home, stops at a store and a day-care center, and continues to work, that is one chain with two stops. In this study, we use the stop-based concept and follow a definition of a trip chain as a sequence of trips bounded by stops of 30 minutes or less. The 30-minute dwell time definition was adopted by the U.S. Federal Highway Administration (FHWA). This is because the various definitions used in previous studies create problems when comparing results. Therefore, we adopt the 30-minute cut-off definition to measure the number of stops between home and work.
Figure 1 shows simple work tour (left) and complex work tour (right). While simple work tour does not have any intermediate destinations, complex work tour has at least one intermediate destination between home and work. This study defines trip-chaining as a complex work tour where non-work activities (i.e., shopping/family errands, medical services, school/daycare/religious activity, social/recreational activity) are conducted on the journey between home and work.
2. The factors influencing trip-chaining
Transportation researchers have recognized that trip-chaining is a common aspect of travel behavior (Adler and Ben-Akiva, 1976). Much of the literature on trip chaining has focused on what factors lead to trip chaining. Some of this research look at how an individual’s characteristics, such as gender (McGuckin and Murakami, 1999; McGuckin et al., 2005), age (Golob and Hensher, 2007; Schmöcker et al., 2010) influence trip-chaining. For example, using the 1995 Nationwide Personal Transportation Survey, McGuckin and Murakami (1999) show that women continue to make more trips to perform household-sustaining activities such as shopping and family errands to a greater extent than men. Women, especially with children in the household, are more likely to chain these household-sustaining trips to the trip to and from work. Schmöcker et al. (2010) examine the trip-chaining complexity of older individuals (aged over 65) in London using the 2001 London Area Travel Survey (LATS). Their finding suggests that older people, on average, make more complex tours compared to those under 65.
Others examine the influence of land use patterns on trip chaining (Wallace et al., 2000; Noland and Thomas, 2007). Noland and Thomas (2007) examine the relationship between patterns of trip-chaining and urban form. Their finding indicates that trip frequency is more significant in those areas with suburban population densities than in rural and high-density urban areas.
Part of the existing literature focuses on travel characteristics such as mode and peak time (Gordon et al., 1988; Hensher and Reyes, 2000; Duncan, 2015). For instance, Gordon et al.’s (1988) research shows that non-work travel accounted for over half of all trips in the AM peak and two-thirds of PM peak trips in metropolitan areas. Hensher and Reyes (2000) estimate the relationship between mode choice, especially the use of public transport, and trip chaining. They find that as individuals move from a simple tour (i.e., home-work-home) to an increasingly more complex tour (i.e., home-school-work-home), the likelihood of using public transport decreases with the increasing number of links in the chain.
This study explores the trip-chaining characteristics and their impact on commute mode choice. This study will contribute to the existing literature as follows. First, while previous studies have mainly examined the factors affecting trip-chaining, most have not explicitly focused on the relationship between trip-chaining and commute mode choice. Therefore, the findings of this study will shed light on whether trip-chaining is a motivator or barrier to sustainable transport. Second, we examine the different patterns in men and women in trip-chaining and commute mode choice. The empirical model results will allow us to understand the different commute behavior of men and women, and how men and women share family responsibilities. Lastly, analysis results based on national data significantly improve our understanding of the impact of trip-chaining on commute mode choice.
Ⅲ. Data, descriptive statistics, and methods
1. Data and descriptive statistics
To understand the trip-chaining behavior nationwide, we utilize detailed individual-level data from the 2017 National Household Travel Survey (NHTS). The NHTS is designed to collect information about daily trips by U.S. residents in all 50 states. Respondents report trip purpose, duration, distance, and travel mode for each trip. These datasets can be linked with the respondent demographic characteristics, household characteristics, and vehicle characteristics (U.S. Department of Transportation, 2017).
Table 1 shows the basic descriptive statistics of our sample used in this study. This gives us a sample size of 64,112 individuals. The analysis is based on individuals who work in the labor market. This study defines trip-chaining as a complex work tour where non-work activities are conducted on the journey between home and work. Based on our data, individuals have 0.39 stops when they go to work or home.
About 89 percent of individuals use driving alone, and about 4 percent use carpool. Public transit users account for 3.2 percent of the sample, and 2.44 percent of individuals use non-motorized modes such as walking and cycling. Multiple modes means that individuals have more than one mode, including driving alone, carpooling, public transit, or walking/cycling while commuting. About 1.5 percent of individuals use multiple mode.
Earlier research states women face more complex daily travel decisions than men (Crane, 2007; Namgung and Akar, 2014). Therefore, we explore trip-chaining characteristics by gender. Figure 1 shows the percentage of making stops and not making stops by gender. As can be seen in Figure 2, women are more likely to make stops than men when they go to work or home. Men and women have more non-work stops after work.
Do the stops people make vary by purpose? The 2017 NHTS offers 20 types of trip purposes, but we can classify them into four types, which account for the largest percentage: 1) drop off/pick up other individuals, 2) buy goods (groceries, clothes, appliances, gas), 3) buy meals (go out for a meal, snack, carry-out), and 4) others. However, the proportions of these purposes greatly vary by gender. Figure 3 shows the percentage of stops by selected purposes by gender.
As can be seen in Figure 3, the aim to drop off/pick up other individuals accounts for the most significant share of making a stop in home-to-work tours. In particular, 59 percent of women stop to drop off/pick up other individuals. In contrast with home-to-work tours, about one-fourth accounts for working-related purposes, but except for that, the similar purposes to the home-to-work tours can explain the stop purposes. Men make a stop to buy goods, and women make a stop to drop off/pick up other individuals on the way home from work.
2. Model Specification (Multinomial Logistic Regression Model)
We employ a multimodal logistic regression model for estimating the determinants of individuals’ commute mode choice as follows:
where, j = 1 (driving alone), 2 (carpooling), 3 (public transit), 4 (walking/cycling), 5 (multimodal mode); Pi = demographic characteristics (age, gender, occupation, work status, and race); Hi = household characteristics (household adults, children, vehicles, and household income); Li = location characteristics (urban, suburban, and rural areas); Ti = commuting distance and number of stops.
After the Collinearity Diagnostics test, the number of household workers and VMT variables were deleted. According to the results of Collinearity Diagnostics, the mean variance inflation factor (VIF) is 2.59, which means there are no multicollinearity issues.
Table 2 presents basic statistics of each travel mode used in the model. The average number of stops between home and work is 1.21 in multimodal mode, followed by 0.39 for driving alone, 0.28 for carpooling, 0.27 for public transportation, and 0.15 for walking/cycling.
Ⅳ. Results and discussion
We employ multinomial logistic regression model for estimating the determinants of individual’s commute mode choice. We report the result of multinomial logistic regression model in Table 3.
The model explains factors associated with an individual’s mode choice, and the reference mode is driving alone. As expected, being older makes individuals more likely to choose driving alone as compared to carpooling, public transit, walking/cycling, and multimodal mode. While women are more likely to choose carpooling than driving alone, women are more likely to choose driving alone rather than public transit and walking/cycling. For instance, women tend to choose carpooling 1.5 times higher than men. Professional job workers are more likely to choose public transit and walking/cycling, and service job workers tend to choose walking/cycling than driving alone as compared to other job workers (reference group). Interestingly, part-time workers are more likely to choose carpooling, public transit, walking/cycling, and multimodal mode rather than driving alone. While being Non-Hispanic White tend to choose driving alone than carpooling, public transit, and multimodal mode, being African American are more likely to choose public transit than driving alone.
For household characteristics, increases in number of adults in households are likely to lead to use of carpooling, public transit, walking/cycling, and multimodal mode than driving alone. As expected, increases in number of children and vehicles are more likely to choose driving alone rather than public transit and walking/cycling. For instance, an increase in the number of children makes choosing public transit 15 percent lower than driving alone.
The residential location-related variables include neighborhood categories (urban, suburban, and rural areas). We find that individuals living in urban neighborhoods are more likely to choose carpooling, public transit, walk/bicycle, and multimodal mode rather than driving alone as compared to the individuals living in suburban neighborhoods (reference group). While individuals living in rural neighborhoods tend to choose driving alone over public transit and walking/cycling, they are more likely to choose carpooling than driving alone compared to those in suburban neighborhoods (reference group). We observe that urban neighborhoods make it possible to attract public transit and walking/cycling than driving alone through residential location variables.
The main purpose of this study is to examine the impact of trip-chaining on commute mode choice. We include the number of stops between home and work as a proxy of trip-chaining. The results show that all else being equal, increases in the number of stops on the journey between home and work are more likely to lead to use of driving alone rather than carpooling, public transit, and walking/cycling. This indicates that trip-chaining can act as a barrier for public transit, non-motorized modes (walk/ bicycle), and carpooling. As expected, an increase in commute distances tends to choose driving alone than carpooling and walking/cycling.
Ⅴ. Conclusion
Individuals’ commute travel behavior has been becoming more complicated as they incorporate multiple activities into a single journey between home and work (McGuckin et al., 2005; Schmöcker et al, 2010). It is a growing phenomenon, but it is considered to potential barrier to sustainable transport because trip-chaining is typically associated with decreased flexibility that attracts car users to switch to public transit and active transportation. However, limited work has been done to take into consideration the impact of trip-chaining on commute mode choice. Therefore, this study explored trip-chaining characteristics and its impact on the commute mode choice using data from the 2017 U.S. National Household Travel Survey (NHTS).
The descriptive analyses focused on trip-chaining characteristics by gender. The results show that women make more non-work trips than men when they go to work and return to home, and men and women have more chained trips after work. For men and women, the aim to drop off/pick up other individuals accounts for the most significant share of making a stop in home-to-work tours. They usually have chained trips to drop off/pick up other individuals, buy meals, and buy goods while commuting.
Key findings from our empirical analyses are as follows. First, trip-chaining can act as a barrier for public transit and active transportation. For instance, increases in the number of stops on the journey between home and work are more likely to lead to use of driving alone rather than carpooling, public transit, and walking/cycling. Second, urban neighborhoods are more likely to make individuals use public transit, walking/cycling, and carpooling as compared to suburban neighborhoods. Third, women are more likely to prefer driving alone over public transit and walking/cycling.
The dominance of automobile usage in travel, especially during periods of high traffic congestion, significantly results in huge social costs and the restriction of sustainable development in cities. U.S. policy requires federal agencies and local governments to reform planning to reduce automobile dependency for sustainable transport (Kwon and Akar, 2022). Therefore, it is important for planners and policymakers to identify opportunities to reduce driving alone and switch to public transit, active transportation (walking/ cycling), and carpooling. Trip-chaining is often seen as a way to reduce the costs of travel because activities can be more efficiently carried out when linked in sequence. However, this study demonstrates that trip-chaining can be a barrier to breaking automobile dependency.
This study provides evidence of factors affecting driving alone rather than other modes. For instance, model results showed that individuals are more likely to drive alone than public transit if they have multiple stops between home and work. Ye et al. (2007) argue that the automobile is considered to provide greater flexibility and convenience as compared to public transit, which is generally constrained with respect to schedules and routes/destinations. Therefore, efforts to make public transit a more attractive option to commuters should be placed on interchange quality and system connectivity, which may improve the convenience of transfer.
Our findings also show that women prefer driving alone over public transit and active transportation. The reason for this can be expected to be that women have more household responsibilities than men. As can be seen in descriptive analyses, their need to combine work with household responsibilities makes it likely that they will make multiple stops on the journey between home and work. This indicates the need to develop policies to better cope with women’s trip-chaining patterns. This can be achieved by better synchronizing gender characteristics and public transit systems. For instance, women’s needs, including preferences, safety, security, and comfort, should be addressed in transportation planning and policies.
As with any research study, this study is not without limitations. This study relies on the 2017 National Household Travel Survey (NHTS) dataset, which lacks socio-psychological variables. Physical and socio-psychological factors likely influence trip-chaining and commute mode choices (Ewing and Handy, 2009). Since NHTS does not offer individuals’ perceptions about modes and neighborhoods, our study is limited to physical aspects. We suggest that future research focus on this to extend our understanding of the relationship between trip-chaining and mode choice. The findings and limitations of this study can bring about specific opportunities for future transportation planning research and policy-making.
This study utilizes U.S. data and studies the U.S. trip-chaining. Therefore, the findings of this study may be different from domestic, especially Gyeonggi-do. For instance, relatively high online activities, delivery services, and working from home can result in a simple work tour. In addition, complicated and limited parking lots and traffic congestion can make driving more difficult for trip chaining. Therefore, future research is needed to focus on domestic case studies and compare them with ours. Nonetheless, this study provides Gyeonggi-do with valuable insights into what factors, including trip-chaining, can be a barrier to public transport and active transportation. This will help to conduct strategies of Gyeonggi-do transportation policy.
References
- Adler, T. J., & Ben-Akiva, M. (1976). “Joint-choice model for frequency, destination, and travel mode for shopping trips”, Transportation Research Record, (569)
-
Akar, G., Chen, N., & Gordon, S. I. (2016). “Influence of neighborhood types on trip distances: Spatial error models for Central Ohio”, International Journal of Sustainable Transportation, 10(3): 284-293.
[https://doi.org/10.1080/15568318.2014.903447]
-
Chen, Y. J., & Akar, G. (2017). “Using trip chaining and joint travel as mediating variables to explore the relationships among travel behavior, socio-demographics, and urban form”, Journal of Transport and Land Use, 10(1): 573-588.
[https://doi.org/10.5198/jtlu.2017.882]
-
Crane, R. (2007). “Is there a quiet revolution in women’s travel? Revisiting the gender gap in commuting”, Journal of the American Planning Association, 73(3): 298-316.
[https://doi.org/10.1080/01944360708977979]
-
Duncan, M. (2016). “How much can trip chaining reduce VMT? A simplified method”, Transportation, 43(4): 643-659.
[https://doi.org/10.1007/s11116-015-9610-5]
-
Ewing, R., & Handy, S. (2009). “Measuring the unmeasurable: Urban design qualities related to walkability”, Journal of Urban Design, 14(1): 65-84.
[https://doi.org/10.1080/13574800802451155]
-
Fan, Y. (2017). “Household structure and gender differences in travel time: spouse/partner presence, parenthood, and breadwinner status”, Transportation, 44(2): 271-291.
[https://doi.org/10.1007/s11116-015-9637-7]
-
Golob, T. F., & Hensher, D. A. (2007). “The trip chaining activity of Sydney residents: a cross-section assessment by age group with a focus on seniors”, Journal of Transport Geography, 15(4): 298-312.
[https://doi.org/10.1016/j.jtrangeo.2006.09.005]
-
Gordon, P., Kumar, A., & Richardson, H. W. (1988) “Beyond the journey to work”, Transportation Research Part A: General, 22(6): 419-426.
[https://doi.org/10.1016/0191-2607(88)90045-3]
-
Hensher, D. A., & Reyes, A. J. (2000). “Trip chaining as a barrier to the propensity to use public transport”, Transportation, 27(4): 341-361.
[https://doi.org/10.1023/A:1005246916731]
-
Kwon, K., & Akar, G. (2022). “Have the gender differences in commuting been shrinking or persistent? Evidence from two-earner households in the US”, International Journal of Sustainable Transportation, 16(12): 1121-1130.
[https://doi.org/10.1080/15568318.2021.1971345]
-
Lee, Y., Hickman, M., & Washington, S. (2007). “Household type and structure, time-use pattern, and trip-chaining behavior”, Transportation Research Part A: Policy and Practice, 41(10), 1004-1020.
[https://doi.org/10.1016/j.tra.2007.06.007]
-
McGuckin, N., & Murakami, E. (1999). “Examining trip-chaining behavior: Comaprison of travel by men and women”, Transportation Resarch Record, 1693(1): 79-85.
[https://doi.org/10.3141/1693-12]
-
McGuckin, N., Zmud, J., & Nakamoto, Y. (2005). “Trip-chaining trends in the United States: understanding travel behavior for policy making”, Transportation Research Record, 1917(1): 199-204.
[https://doi.org/10.1177/0361198105191700122]
-
Namgung, M., & Akar, G. (2014). “Role of gender and attitudes on public transportation use”, Transportation Research Record, 2415(1): 136-144.
[https://doi.org/10.3141/2415-15]
-
Noland, R. B., & Thomas, J. V. (2007). “Multivariate analysis of trip-chaining behavior”, Environment and Planning B: Planning and Design, 34(6): 953-970.
[https://doi.org/10.1068/b32120]
-
Primerano, F., Taylor, M. A., Pitaksringkarn, L., & Tisato, P. (2008). “Defining and understanding trip chaining behaviour”, Transportation, 35(1): 55-72.
[https://doi.org/10.1007/s11116-007-9134-8]
- Rosenbloom, S. (2004). Understanding women’s and men’s travel patterns: the research challenge. Research on Women’s Issues in Transportation. Transportation Research Board of the National Academics, Washington, DC, Chicago, Illinois.
-
Schmöcker, J. D., Su, F., & Noland, R. B. (2010). “An analysis of trip chaining among older London residents”, Transportation, 37(1): 105-123.
[https://doi.org/10.1007/s11116-009-9222-z]
- Strathman, J. G., & Dueker, K. J. (1995). Understanding trip chaining, 1990 NPTS Special Reports on Trip and Vehicle Attributes. Publication FHWA-PL-95-033. FHWA. US Department of Transportation.
- U.S. Department of Transportation (2017). 2017 National Household Travel Survey. (Available at http://nhts.ornl.gov, . Accessed 10 March 2023)
-
Wallace, B., Barnes, J., & Rutherford, G. S. (2000). “Evaluating the effects of travelers and trip characteristics on trip chaining, with implications for transportation demand management strategies”, Transportation Research Record, 1718(1): 97-106.
[https://doi.org/10.3141/1718-13]
-
Ye, X., Pendyala, R. M., & Gottardi, G. (2007). “An exploration of the relationship between mode choice and complexity of trip chaining patterns”, Transportation Research Part B: Methodological, 41(1): 96-113.
[https://doi.org/10.1016/j.trb.2006.03.004]
2022년 미국 The Ohio State University에서 도시및지역계획학 박사학위를 받았다. 논문 제목은 “The Relationship between Socio-Demographic Constraints, Neighborhood Built Environment, and Travel Behavior: Three Empirical Essays” 이다. 현재 미국 캘리포니아 대학교 데이비스 교통연구원에서 박사후연구원으로 재직중이다. 관심분야는 도시 및 지역계획, 토지이용 및 교통, 도시 분석, 스마트 모빌리티 등이다.